
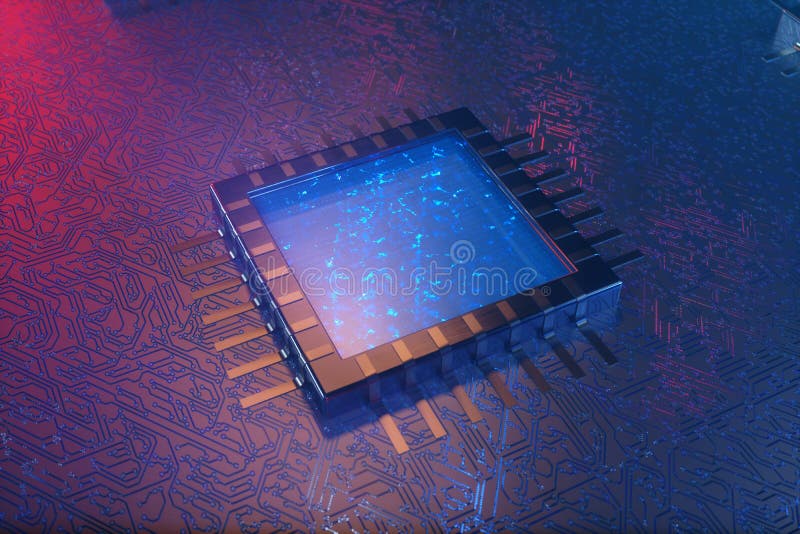
Modern electronic computing is encountering practical limits not only in processing speed but in power consumption, which increases rapidly at high speeds and has forced designers to divide semiconductor processors into multiple cores for operation at more than a few gigahertz.ĭevelopers hope that the combination of optical parallelism, photonic integration, and silicon photonics can bring latency down to meet the demanding limits of deep learning in applications like autonomous vehicles. That makes latency a problem when handling large volumes of data. Driving is a particular challenge because it must be done in real time a self-driving car cannot stop in the middle of the road and wait to identify the unknown thing moving across the road. Success requires processing large volumes of data very quickly. Mathematical processing of many levels of data using tools including matrix-vector multiplication, convolution, and Fourier processing has brought success in applications including speech recognition, image classification, and driving autonomous vehicles.
#Luminous computing ai bill skin#
For example, a neural network may use multiple factors to recognize a face, such as nose shape, hair color and pattern, eye color, skin color, space between the eyes, and positions of other facial features. Humans are more adaptable.ĭeep learning expands on machine learning by sorting through larger data sets using neural networks with multiple levels of processing. An AI chess champion couldn’t drive a car around the block, and a self-driving car AI would not know what to do about a large red truck with hoses attached and light flashing that is stopped in the road unless it had previously learned about a fire truck doing all those things. Yet AIs must be trained for specific tasks, and can’t recognize other things not in the training set. An AI performs brilliantly at games with well-defined rules that it has played many times it can beat human champions, whom we consider highly intelligent. You might think of an AI as an alien mind, and it has different skills than humans. Images often are a starting point, but the systems analyze other types of data as well. 1 A machine-learning system learns by processing input information using special algorithms designed to recognize patterns in the data. In machine learning, “the core computational algorithm is not fully provided by a programmer, but automatically generated or improved by a computer system through experience,” wrote Qixiang Cheng, now at the University of Cambridge (Cambridge, England), in a review article. An ‘alien’ intelligenceĪlthough neural networks inspired the design of machine learning, AI does not work like the human mind. Developers are looking to silicon photonics to provide the needed boosts of speed and power. However, deep learning requires processing huge volumes of information using complex processes such as matrix vector manipulation, and electronic computers cannot keep up with the rapidly increasing demands. Machine-learning systems have found applications ranging from filtering spam from your email to recommending films on Netflix, but their most famous uses are beating humans at complex games such as Go and chess.ĭeep learning extends on machine learning by using more-complex neural networks to tackle more-complex tasks, including speech recognition and autonomous driving. Its revival came from machine learning, which programs computers to gather and analyze large volumes of information using neural networks, which like the human brain have massive interconnections among their processors. Like optical computing, AI was slow getting off the ground, and suffered an “AI Winter” in the 1980s and early 1990s. Isaac Asimov’s famed robot stories inspired Marvin Minsky, who as a young professor launched the MIT Artificial Intelligence Laboratory in the early 1960s. AI’s roots literarily came from science fiction. Now that semiconductor features have shrunk to the nanometer scale and Moore’s law is running out of room, a new generation of integrated photonics could boost the speed and processing power of artificial intelligence (AI) beyond what electronics can provide.ĪI has its own long history of promises that have proved hard to fulfill. In the 1980s, the late John Caulfield told me how a new generation of optical computing could do things we could never imagine, but couldn’t balance your checkbook yet again, electronics proved faster, better, and cheaper.
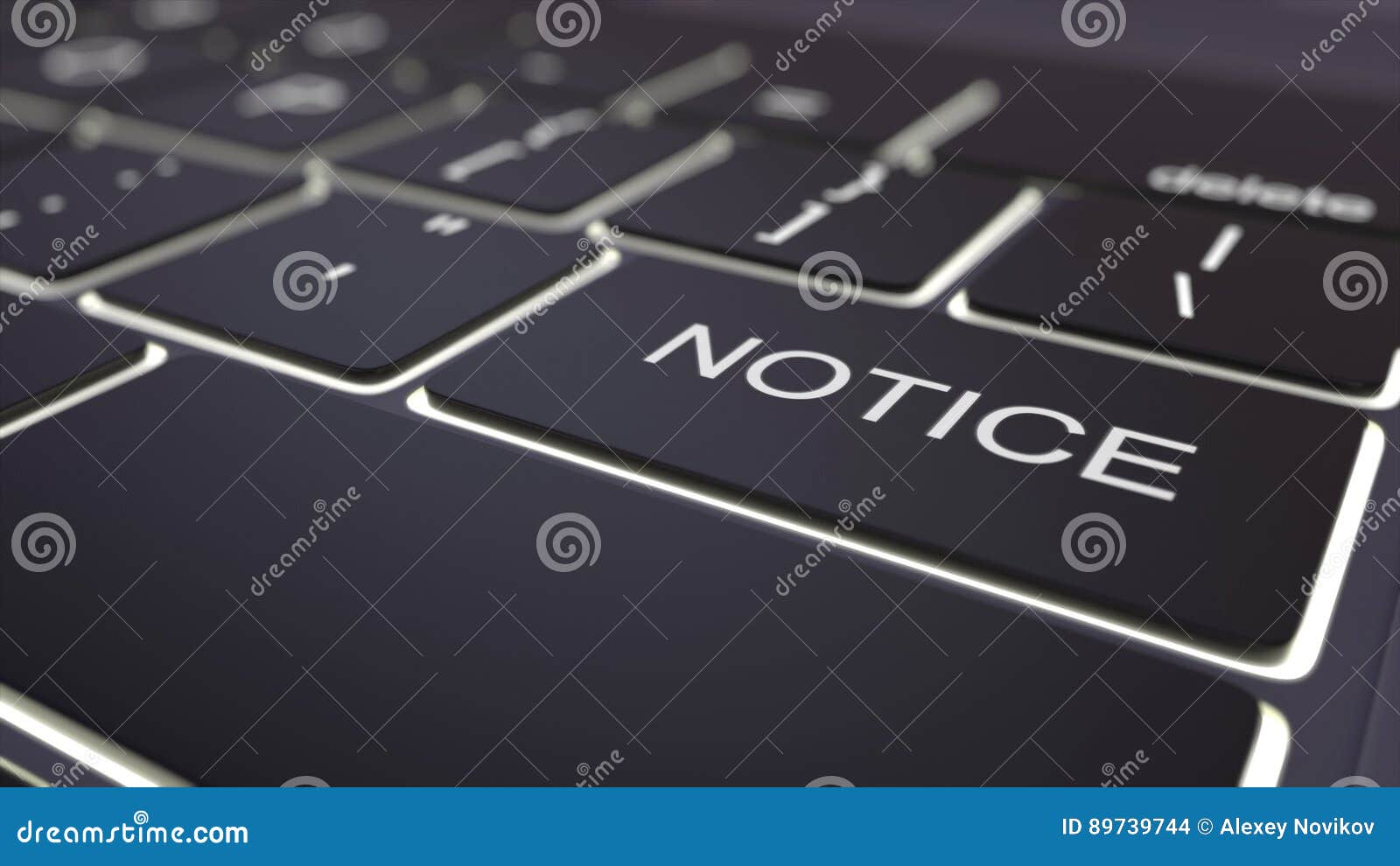
Optical processing that generated military maps by Fourier transforms of synthetic-aperture radar data were so successful in the 1950s that they were classified, although electronic fast Fourier transforms eventually won out. Old-timers know optical computing is not all new.
